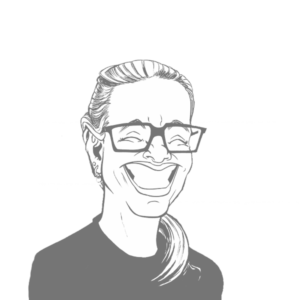
Kylie Willett
Director, Design and Product
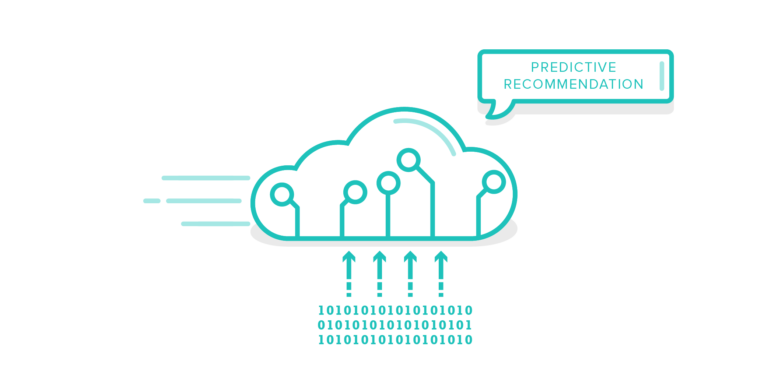
AI is now within reach
Businesses commonly bemoan data quality and business processes when the prospect of deploying Artificial Intelligence (AI) comes up. Here at Lightfold, we’ve got a very different opinion on your data assets and ways around the potential mire that is DQM (data quality management).
Working with a wide variety of business, both large and small, Lightfold has been a proud participant in the ascendance of tactical AI. This ascendance has been propelled in no small way by ingenious productization across the industry. Predictive analytics is now being democratised by tools like Einstein Discovery, in the same fashion that the proliferation of BI (business intelligence) tools broadened the use and consumption of descriptive analytics. Rather than costly, specialist endeavours, predictive analytics is now within easy reach for most businesses.
“The democratisation of AI and predictive analytics has shifted old problems, like DQM, into a new light, as well as creating entirely new issues.”
Not all data is created equal
The key here is understanding how AI relies on data, and that that not all data is AI-ready. We’ve all seen the stats about the explosion of data in the last decade, and it’s predicted that by 2025, 463 exabytes of data will be created globally every day. Building AI models is rapidly becoming a totally unremarkable business activity, but making the vast lakes of underlying data ready for use in AI is still something of a challenge.
“Creating AI that drives real business outcomes like retaining customers, growing sales or deepening key relationships, lives and dies on the underlying data being both concrete and actionable.”
What do we mean by this? Put simply, concrete data refers to things where there is little or no abstraction, for example ‘The customer lives in Brisbane’ or ‘The customer has bought $300 worth of product A’. In comparison, highly abstracted data generated by business processes offers little predictive value. Actionable data is data that can be changed by the actions of users. A customer’s birthday is unlikely to be something your end users could change, but the duration since their last meeting with the customer can absolutely be changed – by making an appointment to see them!
“Concrete data refers to things where there is little or no abstraction. Actionable data is data that could be influenced or changed by the actions of your users.”
AI readiness is all about making it real
It’s this concept that is not always obvious to businesses when they consider their readiness for AI. When businesses complain about their DQM, they are often speaking more about the patchiness of the abstracted business process data. Those missing fields from half the sales rep’s meeting reports are not going to be a blocker. In our experience, we almost always find a trove of useable data assets despite the more top of mind concerns about how DQM and business processes fail to line up. If you’re questioning whether your data can meet the requirements of a predictive project, the question you should consider is: how much do your data assets reflect real aspects of your customers and your products and services?
At Lightfold we tend to be quite sceptical of the idea that problems with data quality can completely block an AI project. Competition is high, customer expectations are even higher and the current situation with COVID-19 is turning those screws even tighter. We will often say to clients: “If you’re not using your crap data – someone else will!”. It’s a flippant line but it has a deliberate, deeper message:
“There is an opportunity to squeeze value out of your data assets very quickly with AI. Concerns about data quality shouldn’t stop you from taking advantage of that opportunity.”
Data enrichment is a shortcut to quick wins
Now we know that AI is hungry for concrete and actionable data, what avenues are apparent for us to boost the value and speed of predictive analytics projects? At a strategic level it suggests an immediate broad objective for the business data model, a reorientation to real data objects and a paring back of abstracted data collection. At Lightfold, we call this the AI First Strategy (Watch this space for more on the AI First Strategy!). From a tactical perspective it means that data enrichment is king. Expanding the scope of your data that meets AI requirements is a fast way to readily expand the potential use cases of predictive analytics. Put simply, the more you know, the more you can predict, and the better your predictions will be.
Like DQM, data enrichment is often chucked into the ‘too hard’ basket. And historically this has been pretty reasonable for a market like APAC, where we just don’t have the scale of other economies like the US or EU. That’s all about to change. Lightfold has partnered with Precisely, who are the recently rebranded Pitney-Bowes Syncsort, an entity with an impeccable 30-year heritage in data handling, processing and transformation. Working together, Lightfold and Precisely are bringing new data products to market that provide quick wins for businesses looking to expand their customer data footprint.